Neural-Mechanistic hybrid modelling improve microorganism phenotype predictions, Nature communications
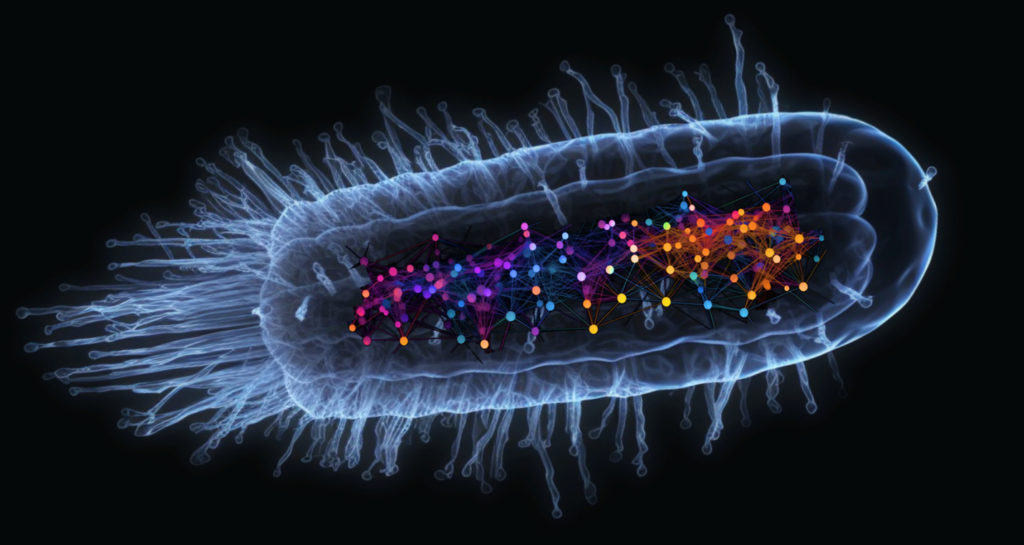
Constraint-based metabolic models have been used for decades to predict the phenotype of microorganisms in different environments. However, quantitative predictions are limited unless labor-intensive measurements of media uptake fluxes are performed. We have recently showed how hybrid neural-mechanistic models can serve as an architecture for machine learning providing a way to improve phenotype predictions. We have illustrated the hybrid approach with growth rate predictions of Escherichia coli and Pseudomonas putida grown in different media. We have also used our hybrid models to predict phenotypes of gene knocked-out Escherichia coli mutants.
Our neural-mechanistic models systematically outperform traditional constraint-based models and require training set sizes orders of magnitude smaller than classical machine learning methods. The proposed novel hybrid models open a doorway to enhancing mechanistic modeling: instead of constraining mechanistic models with additional experimental measurements, the hybrid approach grasps the power of machine learning while fulfilling mechanistic constraints, thus saving time and resources in typical systems biology or biological engineering projects.
Ref : Faure, L., Mollet, B., Liebermeister, W. et al. A neural-mechanistic hybrid approach improving the predictive power of genome-scale metabolic models. Nat Commun 14, 4669 (2023). https://doi.org/10.1038/s41467-023-40380-0